Generative AI is the ability of a computer program to create brand-new, completely original variations of content (including images, video, music, speech and text). Generative AI (GenAI) can analyze, improve or alter existing content, and it can create new data elements and novel models of real-world objects, such as buildings, parts, drugs and materials.
GenAI has the potential to significantly impact shareholder value by providing new and disruptive opportunities to increase revenue, reduce costs and improve productivity, and better manage risk. In the near future, GenAI will become a competitive advantage and differentiator.
GenAI capabilities will increasingly be built into the software products that companies and customers have likely already purchased and use everyday, such as OpenAI, Google Bard, Bing, Microsoft 365, Microsoft 365 Copilot and Google Workspace. This is effectively a “free,” or close to free, tier with benefits to productivity that will naturally flow to the organization. Vendors will pass costs on to customers as part of bundled incremental price increases for both GenAI and other capabilities they build into their tools.
GenAI market is composed of the four segments
GenAI is not a market per se; it permeates across the entire technology stack and the majority of verticals. The new way to interface with technology is bringing disruption to the technology usage patterns for both consumers and workers.
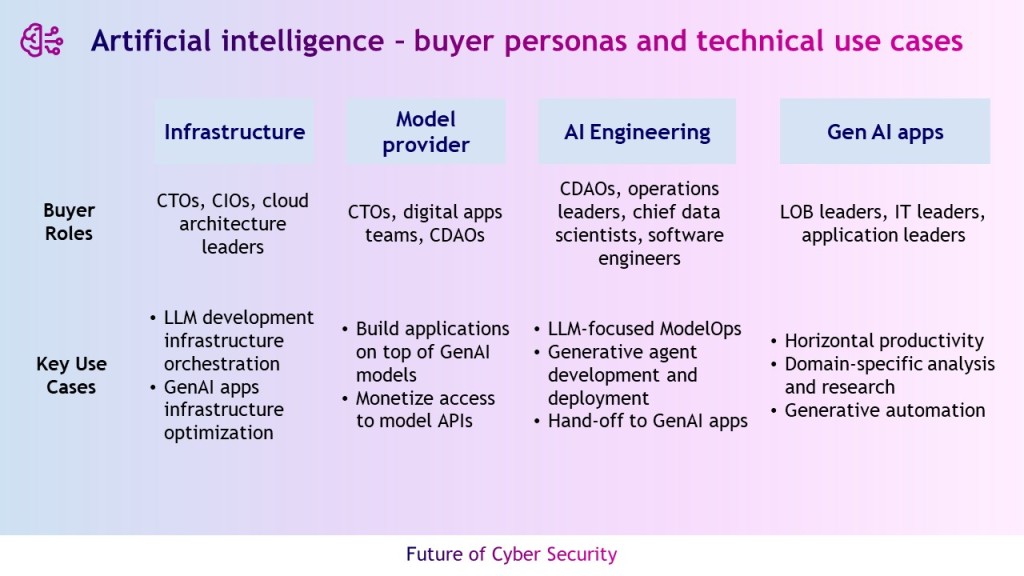
- Infrastructure providers — This segment comprises the vendors of infrastructure (both hardware and IaaS and PaaS offerings) to support the GenAI needs for compute, storage and network. It also includes software providers offering capabilities for infrastructure orchestration, tuning and scaling for GenAI development and applications in production.
- Model providers — This layer of vendors offers access to commercial or open-source foundation models such as LLMs and other types of generative algorithms (such as GANs, genetic/evolutionary algorithms or simulations). These models can be provided for developers to embed into their applications or be used as base models for fine-tuning customized models for their software offerings or internal enterprise use cases.
- AI engineering — The vendors in this segment are made up by incumbent and startup vendors covering full-model life cycle management, specifically adjusted to and catering to development, refinement and deployment of generative models (e.g., LLMs) and other GenAI artifacts in production applications.
- Generative AI apps — GenAI applications use GenAI capabilities for user experience and task augmentation to accelerate and assist the completion of a user’s desired outcomes. When embedded in the experience, GenAI offers richer contextualization for singular tasks such as generating and editing text, code, images and other multimodal output. As an emerging capability, process-aware GenAI agents can be prompted by users to accelerate workflows that tie multiple tasks together.
Security leaders to protect their organizations GenAI
A proliferation of overoptimistic generative AI (GenAI) announcements in the security and risk management markets could still drive promising improvements in productivity and accuracy for security teams, but also lead to waste and disappointments.
Consumption of GenAI applications, such as large language models (LLMs), from business experiments and unmanaged, ad hoc employee adoption creates new attack surfaces and risks on individual privacy, sensitive data and organizational intellectual property (IP).
Many businesses are rushing to capitalize on their IP and develop their own GenAI applications, creating new requirements for AI application security.
Attackers will use GenAI. They’ve started with the creation of more seemingly authentic content, phishing lures and impersonating humans at scale. The uncertainty about how successfully they can leverage GenAI for more sophisticated attacks will require more flexible cybersecurity roadmaps.
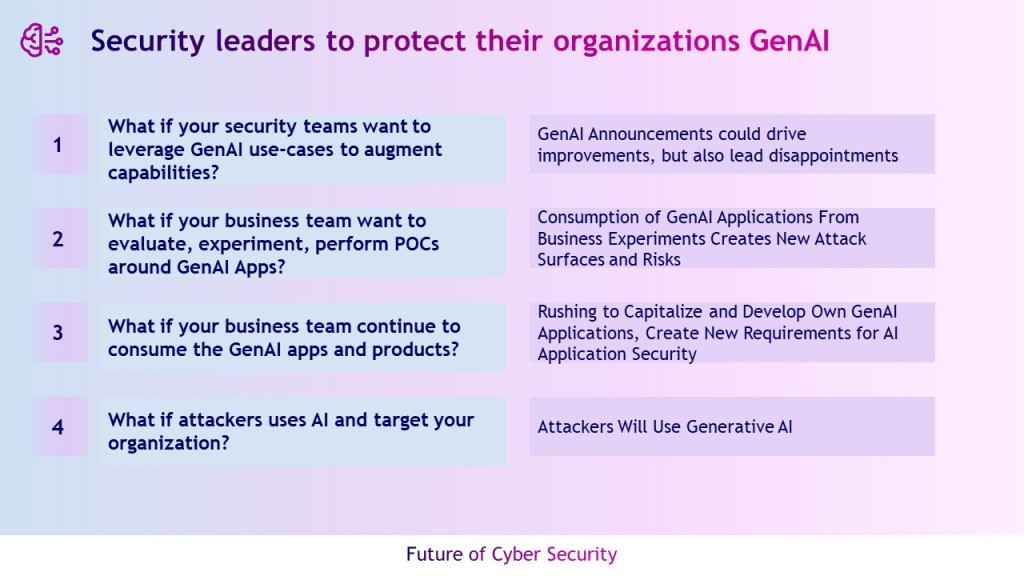
CISOs need to be empowered addressing below GenAI impacts
- What if my security team want to leverage GenAI use-cases?
- What if my business team want to evaluate, experiment, perform POCs around GenAI apps?
- What if my business team continue to consume the GenAI products?
- What if my organizations targeted by AI attacker?
Guidance for security leaders in GENAI initiatives
To address the various impacts of generative AI on their organizations’ security programs, chief information security officers (CISOs) and their teams must:
- Run experiments starting with targeted and narrow use cases in the security operation and application security areas
- Establish or extend existing metrics to benchmark to measure expected productivity improvements
- Identify changes in data processing and supply chain dependencies and require transparency about data usage
- Adapt documentation and traceability processes to ensure internal knowledge augmentation and avoid only feeding the tools with your insights.
- Choose fine-tuned or specialized models that align with the relevant security use case or for more advanced teams.
- Prepare and train your team for dealing with direct (privacy, IP, AI application security) and indirect impacts (other teams using GenAI, such as HR, finance or procurements) coming from generative AI uses
- Upskill talents DeepLearning.AI is an education technology company that is empowering the global workforce to build an AI-powered future through world-class education, hands-on training, and a collaborative community.
- Apply AI TRiSM principles AI trust, risk and security management (AI TRiSM) ensures AI model governance, trustworthiness, fairness, reliability, robustness, efficacy and data protection. This includes solutions and techniques for model interpretability and explainability, AI data protection, model operations and adversarial attack resistance.
- Consider the data security options when training and fine-tuning models, notably the potential impact on accuracy or additional costs. This must be weighed against requirements in modern privacy laws, which often include the ability for individuals to request that organizations delete their data.
- Adapt to hybrid development models, such as front-end wrapping (e.g., prompt engineering), private hosting of third-party models and custom GenAI applications with in-house model design and fine-tuning
Leave a comment